The Role of AI in E-commerce Optimization
The Role of AI in E-commerce Optimization
This year, e-commerce sales will surpass 5.7 trillion dollars by the end of 2022. Companies compete to capitalize on increased online shopping demand by improving the user shopping experience. Yet, nothing has more profoundly influenced consumer behavior than the role of AI in e-commerce optimization.
AI-based recommendation algorithms have been the key driver in e-commerce adoption. Google Play estimates that 40% of its app installs came from recommendations!
Traditional e-commerce optimization methods work but are not always as efficient as their AI counterpart. Either method relies on correct product placement to increase consumer sales goals.
Learn how AI e-commerce optimization can help your online business succeed in this article.
Key Takeaways
- Businesses must market e-commerce products to the correct demographic
- Traditional e-commerce optimization methods are not always as efficient as their AI counterpart
- AI can determine the relatedness of different items to anticipate user behavior and generate efficient recommendations
The Goal of E-commerce Optimization
As mentioned, to succeed in e-commerce, you must market products to the correct demographic. People are more likely to buy when the product is relevant.
For example, you might have gone apartment hunting or house shopping and had to weigh the pros and cons of different factors that depend on your needs. You might have considered the home’s amenities, location, or size. You might even have had a housing coordinator or realtor recommending different housing options based on your preferences.
Unlike traditional brick-and-mortar, you only have a web page to help when you’re online. If the store is not optimized to deliver relevant products based on your queries, you will likely become another abandoned cart statistic. The e-commerce storefront has the burden of optimizing results to be relevant, regardless of the user.
The Role of Manual-Based Recommendations in E-commerce Optimization
E-commerce has tried to navigate the shortfalls of dropped checkouts with recommendation retargeting models that rely on manual input. Popular manual recommendations use “tags” or sales history.
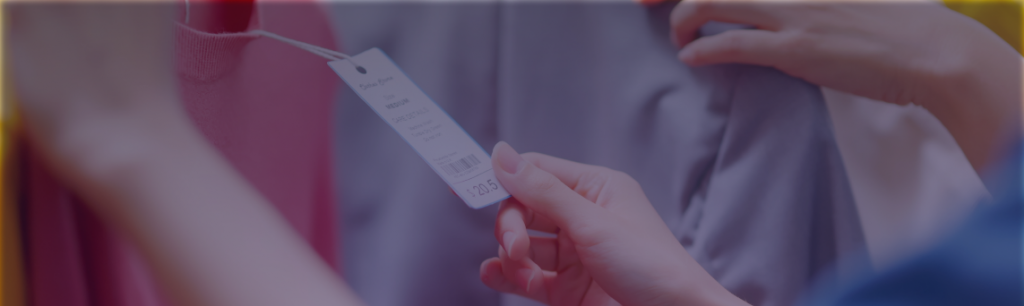
If we imagine a clothing storefront, a clothing store can “tag” clothing items by size, length, and color and then return short lists of related items that meet your criteria. Stores can also organize results based on sales history and make reasonable assumptions as to what products they might prefer based on the sales purchases of others.
Legacy History Models are Not Effective
Unfortunately, the sales history method is inefficient because unwanted “noise” can reduce the quality of recommendations. New products will have no data to base recommendations on, and popular items might be recommended simply for being bestselling, not because they are relevant to the user. Tag-based systems have a different Issue.
Tag Optimization Models are Time Consuming
Tag-based systems are time-consuming. Let’s take Amazon, for example, which has over 10 million customers worldwide, over 150 million square feet of storage space, and millions of products. Could you imagine the time needed to tag more than a million Items? Nothing short of a nightmare!
Feature-rich products magnify the up-front work needed to design tag-based optimization models. As mentioned in this popular data science competition, the housing problem mentioned earlier has over 60 features you might consider relevant to your buying decision. Imagine having to tag 1 million items with 60 individual features.

Not an impossible task, but a laborious one if you have thousands of items in your store index with 100s of features. But it isn’t easy to implement in stores that have seasonal products or items that are regularly updated.
Tag-based systems also require guesswork on what the user will want, with many reliant on the user’s ability to query their item properly, which is not always the case. If the user searches for items with nonexistent tags, they might get no meaningful recommendations from the store. Guessing such tags also eats the greatest resource of all time – your time.
The Role of AI in E-commerce Optimization
Ever wonder how YouTube or Amazon can keep making magical suggestions? AI saves time and increases the relevancy of recommendations by leveraging user data to make predictions. Rather than guess, AI can determine the relatedness of different items to anticipate user behavior and generate recommendations. Recommendation engines can even offer suggestions unknown to the consumer!
AI Under the Hood
AI recommendations take a consumer corpus, a collection of organized data sets, and apply algorithms (with fancy names such as decision forest trees and xboost) to generate predictive models that your storefront can utilize by programmatic requests via an API or an app to a cloud-based service. The AI then sends results back, and your store can generate product results in real-time for supercharged product recommendations.
AI Optimization in Action
As mentioned, various models can be used to formulate predictions for the best product placement. Collaborative filtering and content-based filtering algorithms are some of the most useful when it comes to predictive models. We typically use click streams and conversions to populate our data models that make predictions based on the consumer journey and their goals.
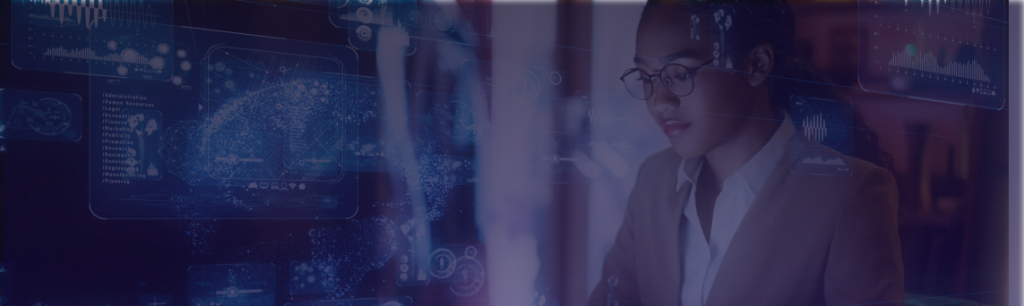
An AI Role Example
Let’s suppose we visit an e-commerce movie store that AI powers. Let’s say I only like horror movies, and Customer B only enjoys children’s movies, and then we have Customer C, who watches both genres and falls into neither category.
We first start by having AI process our data features, discovering that Customer C enjoys blockbusters, which is why they fell into both categories.
Suddenly, we get Customer D, who only likes blockbusters and horror movies but not children’s films. So far:
- I only like Horror Movies
- Customer B – Only likes children’s movies
- Customer C – Only likes blockbusters
- Customer D – Likes all blockbusters and horror movies, but not children’s movies
Our collaborative-filtering AI would then give recommendations based on our mutual interests. For example, if a new Sci-fi-Horror movie came out, and I liked the movie, the AI would not be familiar with the Sci-fi classification but would show the recommendation to Customer D because we are similar.
Likewise, the AI would not show the result to Customer B because they only like children’s movies. Customer C might then see the movie to determine if it is a blockbuster.
The result is an AI that is not only making better predictions but an AI that is also learning. No longer are we forced to display product results that can only process individual events but instead use complex click streams that follow the entire consumer journey.
Let AI Transform Your E-commerce Optimization
AI uses user data to outperform manual-based optimizations that take time and are inefficient. Manual-based optimization attempts can still work but might be unnecessarily taxing and unreliable, especially in large, dynamic product collections. Why take the risk of losing extra sales?
To learn more and request a demo, contact Hypersonix today.