What Are the Top Challenges in Implementing AI-driven Analytics Solutions?
What Are the Top Challenges in Implementing AI-driven Analytics Solutions?
Businesses are increasingly turning to AI-driven analytics to gain competitive insights and make informed decisions. However, the journey to successfully implementing these advanced analytics solutions is fraught with challenges.
From data quality issues to the complexities of scaling AI systems, organizations must address a myriad of obstacles to fully utilize AI-driven analytics. This article explores the top challenges in implementing AI-driven analytics solutions and provides insights into how businesses can overcome them to unlock their full potential.
Quick Takeaways
- Organizations must overcome issues like data silos, inconsistencies, and inaccuracies to ensure reliable AI analytics.
- Effective AI implementation requires scalable infrastructure and solutions to manage increasing data volumes without performance bottlenecks.
- Addressing the shortage of skilled professionals in AI and data analytics is crucial for successful deployment and management of AI solutions.
- Robust data protection measures and compliance with regulations are essential to maintaining trust and ensuring the ethical use of AI-driven analytics.
1. Data Quality and Integration
Organizations often struggle with data silos, inconsistent data formats, and the sheer volume of data, which can impede accurate analysis. Poor data quality can lead to inaccurate or biased AI models, affecting decision-making and business outcomes. Incomplete, inaccurate, outdated, duplicate, and inconsistent data are common issues that organizations must address to achieve reliable AI analytics.
A report by Precisely and Drexel University’s LeBow College of Business found that while 77% of data and analytics professionals aim for data-driven decision-making, only 46% trust the data used for these decisions.
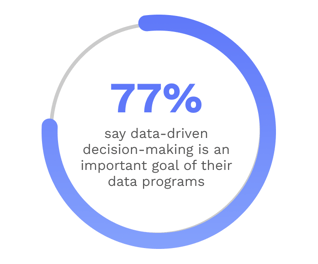
This highlights the significant challenge of data quality in AI analytics implementations. To mitigate these challenges, organizations should establish:
- Robust data collection procedures
- Regular data cleaning
- Data validation rules
Ensuring data is up-to-date and performing routine data quality audits are also essential steps. Additionally, integrating data from multiple sources carefully can prevent data conflicts and loss of integrity.
2. Scalability Issues
As data volumes grow, the complexity of processing and analyzing it increases. Many organizations find their existing infrastructure inadequate for handling large-scale AI deployments, leading to performance bottlenecks and inefficiencies. Ensuring that AI systems can scale efficiently requires substantial investments in infrastructure and continuous optimization.
To overcome scalability issues, organizations must invest in scalable infrastructure and leverage cloud-based solutions that offer flexibility and capacity on demand. Employing advanced algorithms that can handle large datasets efficiently and using data management practices that ensure smooth scalability are also critical strategies.
3. Skill Gaps and Training
The lack of skilled professionals to implement and manage AI solutions is another major hurdle. AI-driven analytics requires specialized knowledge in:
- Data science
- Machine learning
- Advanced analytics techniques
Many organizations face difficulties in finding and retaining talent with the necessary expertise, which can delay or hinder the successful deployment of AI projects.
A Gartner survey revealed that less than half (44%) of data and analytics teams are considered effective in providing value to their organizations, underlining the need for enhanced training and skill development to support AI-driven analytics.
Bridging the skill gap involves continuous training and development programs for existing staff and hiring new talent with the required skills. Organizations should also consider partnerships with educational institutions and online learning platforms to provide ongoing training and certification programs. Creating a culture that encourages learning and staying updated with the latest advancements in AI and analytics can help address this challenge effectively.
4. Cost and ROI Concerns
Implementing AI-driven analytics solutions can be costly, involving significant upfront investments in:
- Technology
- Infrastructure
- Skilled personnel
Many organizations struggle with justifying these expenses, especially when the return on investment (ROI) is not immediately apparent. High initial costs can be a deterrent, particularly for small and medium-sized enterprises (SMEs).
Businesses lose $3 trillion annually due to poor data quality, highlighting the severe financial impact of bad data on organizations. This significant loss emphasizes the importance of investing in high-quality data management to avoid costly errors and inefficiencies.
To address cost and ROI concerns, organizations should conduct thorough cost-benefit analyses before embarking on AI projects. This includes estimating the potential financial gains from:
- Improved efficiency
- Better decision-making
- Enhanced customer experiences
Leveraging cloud-based AI solutions can also help reduce infrastructure costs by offering scalable and flexible pricing models. Moreover, starting with pilot projects to demonstrate value and build a business case for broader AI adoption can help secure executive buy-in and funding.
5. Data Privacy and Security
Data privacy and security are paramount concerns when implementing AI-driven analytics solutions. Organizations must ensure that sensitive data is protected from breaches and unauthorized access.
Compliance with data protection regulations, such as GDPR and CCPA, adds another layer of complexity. Ensuring that AI systems handle data ethically and transparently is crucial to maintaining customer trust and avoiding legal repercussions.
To mitigate these challenges, organizations should implement robust data encryption methods and access controls. Regular security audits and updates to security protocols can help protect against emerging threats.
Additionally, adopting privacy-by-design principles, where privacy considerations are integrated into the AI development process from the beginning, can ensure compliance and build trust with stakeholders. Providing clear and transparent communication about data usage and privacy policies is also essential.
6. Change Management
Implementing AI-driven analytics solutions often requires significant changes in organizational processes and culture. Resistance to change is a common challenge, as employees may fear job displacement or struggle to adapt to new technologies. Effective change management is crucial to ensuring the successful adoption of AI solutions and realizing their full potential.
Organizations should focus on fostering a culture of innovation and continuous improvement. This involves clear communication about the benefits of AI and how it can enhance, rather than replace, human roles.
Providing comprehensive training and support can help employees develop the necessary skills and confidence to work with AI technologies. Involving employees in the AI implementation process and encouraging their input can also reduce resistance and promote a sense of ownership.
Unlock the Full Potential of AI-Driven Analytics with Hypersonix
Successfully implementing AI-driven analytics solutions requires addressing several significant challenges. By understanding and proactively tackling these obstacles, organizations can unlock the full potential of AI-driven analytics and gain a competitive edge in their industries.
Discover how Hypersonix’s AI-driven analytics solutions can help you overcome data quality, scalability, skill gaps, and security challenges. Book a demo today to unlock the full potential of AI in your organization and drive successful decision-making!