Demand Planning Vs Forecasting: How Are They Different?
Demand Planning Vs Forecasting: How Are They Different?
Accurate demand planning and forecasting can make the difference between a well-oiled supply chain and costly inefficiencies. As businesses strive to meet customer expectations and manage resources effectively, understanding these two essential processes becomes crucial.
In this article, we will clarify the differences between demand planning and forecasting, exploring how they complement each other and how leveraging both can optimize supply chain operations. By examining key components, methodologies, and the role of technology, this article will clarify the distinctions and synergies between these vital processes.
Quick Takeaways
- Demand planning relies on historical sales data, market trends, and statistical analysis to predict future customer demand accurately.
- The primary components of demand planning include data collection, demand analysis, and demand forecasting.
- Technology, especially AI and machine learning, enhances demand planning by improving data accuracy and forecasting precision.
- Forecasting uses quantitative and qualitative methods to predict future demand based on historical data and analysis.
Understanding Demand Planning
Demand planning is a crucial process in supply chain management that involves predicting future customer demand to ensure that products are available when needed. This process relies heavily on historical sales data, market trends, and statistical analysis to forecast demand accurately.
The primary components of demand planning include:
- Data collection: Involves gathering relevant information from various sources, such as sales records, market research, and customer feedback.
- Demand analysis: Interprets this data to identify patterns and trends.
- Demand forecasting: Uses statistical models to predict future demand based on the analyzed data.
Technology plays a significant role in demand planning by providing advanced tools and software that enhance data accuracy and forecasting precision. The integration of big data analytics and machine learning has significantly improved the accuracy of demand forecasts. These technologies help analyze large datasets, identifying complex patterns and making precise predictions.
Understanding Forecasting
Forecasting, on the other hand, focuses on predicting future events based on historical data and analysis. In the context of supply chain management, forecasting aims to anticipate future demand to ensure that resources are allocated efficiently and customer needs are met promptly.
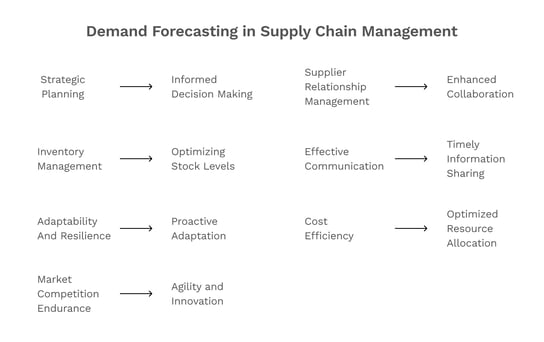
The global recession, high inflation, and shifting consumer preferences have complicated demand forecasting. Retailers are witnessing a decline in demand for premium products, and many buyers are focusing more on necessities.
Forecasting techniques can be broadly categorized into quantitative and qualitative methods. Quantitative forecasting uses mathematical models and historical data to predict future demand. Common techniques include:
- Time series analysis
- Regression models
- Econometric models
Qualitative forecasting, meanwhile, relies on expert judgment, market research, and subjective analysis to make predictions.
Historical data is a cornerstone of forecasting, providing the basis for statistical analysis and model development. Predictive analytics, enhanced by AI and machine learning, further refines forecasting accuracy by identifying hidden patterns and trends that may not be apparent through traditional methods.
Key Differences Between Demand Planning and Forecasting
While demand planning and forecasting are interrelated, they serve distinct purposes within supply chain management. Understanding these differences is key to leveraging both processes effectively.
Objectives
Demand planning is strategic and long-term, aiming to create a comprehensive plan that aligns supply with anticipated demand. In contrast, forecasting is more tactical and short-term, focusing on predicting future demand based on historical data and current trends.
Methodologies
Demand planning uses a holistic approach, integrating various data sources and business insights to develop a robust supply chain strategy. It involves cross-functional collaboration and scenario planning. Forecasting, however, primarily relies on statistical methods and quantitative analysis to predict future demand, often using specific models like time series analysis or regression.
Data Usage
Demand planning incorporates a wide range of data, including:
- Sales history
- Market research
- Customer feedback
It also considers external factors such as economic conditions and competitor actions. Forecasting, on the other hand, is heavily dependent on historical sales data and statistical algorithms to generate predictions.
Outcomes
The outcome of demand planning is a detailed action plan that guides:
- Inventory management
- Production schedules
- Procurement strategies
It helps businesses ensure they have the right products in the right quantities at the right time. Forecasting, meanwhile, provides the predictive insights that inform these plans, helping to anticipate demand fluctuations and adjust strategies accordingly.
Integrating Demand Planning and Forecasting for Optimal Results
Integrating demand planning and forecasting is crucial for achieving optimal supply chain performance. These processes complement each other, providing both strategic direction and predictive accuracy.
Demand Planning Benefits from Forecasting Insights
Demand planning benefits from the predictive insights generated by forecasting, allowing businesses to create more accurate and responsive supply chain strategies. For example, a robust demand forecast can inform production schedules, inventory levels, and procurement plans, ensuring that resources are allocated efficiently and customer demand is met promptly.
According to a recent study, 9 out of 10 organizations reported measurable value from their data and analytics investments in 2023, highlighting the significant impact of accurate demand forecasting on business performance.
Despite these benefits, many businesses are still in the process of upgrading their technological capabilities. As of 2023, 73% of supply chain leaders still use spreadsheets for planning and forecasting.
However, 90% have prioritized technology upgrades and are taking steps toward innovation adoption. Among the technologies these organizations plan to adopt, machine learning and demand forecasting are top priorities for 80% of supply chain executives.
.
Forecasting Enhanced by Demand Planning
Forecasting, in turn, is enhanced by the comprehensive approach of demand planning. By incorporating data and business insights, demand planning helps to refine forecasting models and improve their accuracy. This iterative process ensures that forecasts remain relevant and aligned with business objectives.
Seeking Integrated Solutions
To maximize the benefits of integrating these processes, businesses should seek solutions designed to support both demand planning and forecasting. Advanced tools that offer enhanced data accuracy, predictive analytics, and decision-making capabilities can significantly improve the efficiency and responsiveness of supply chain operations.
Use Hypersonix Today for Superior Supply Chain Management
Effective demand planning and forecasting are essential for maintaining a responsive and efficient supply chain. By understanding the key differences and integrating these processes, businesses can optimize their inventory management and resource allocation. Leveraging advanced forecasting techniques and tools can further enhance the accuracy and reliability of demand planning, ensuring success in meeting customer demands.
Discover how integrated demand planning and forecasting solutions can enhance your supply chain efficiency and accuracy. Book a demo with Hypersonix today to see how our AI-driven tools can help you achieve optimal results.