Price Optimization in the AI Era: Retailers Pricing Guide in the 2020s
Price Optimization in the AI Era: Retailers Pricing Guide in the 2020s
Success in retail requires the careful orchestration of pricing, promotions, product assortment and inventory availability. To say that price optimization is anything less than a top priority for retailers would be an understatement. Pricing is perhaps the biggest factor to be able to impact revenue and profits quickly. As Rafi Mohammad, a renowned pricing expert, writes in an Harvard Business Review article, the beauty of focusing on pricing is its immediate effect: Prices can be changed on Sunday evening, and new profits start rolling in on Monday morning.
Price Optimization today comes from a long line of iterations in concept and practice over the years, with the ever-consistent change in consumer behavior.
In this piece, we will look at:
- Why price optimization continues to take center stage time and again
- The evolution of retail pricing and how retailers have adapted
- How retailers can compete in today’s market with AI-driven Autonomous Pricing
Let us begin.
What is price optimization? Why is it important?
In simple terms, price optimization is understanding in advance how customers respond to pricing and price changes while optimally meeting business’ revenue and profit objectives. More clearly, if lower prices are presented to the customer, will they buy more? If higher prices are presented to the customer, will they buy less?
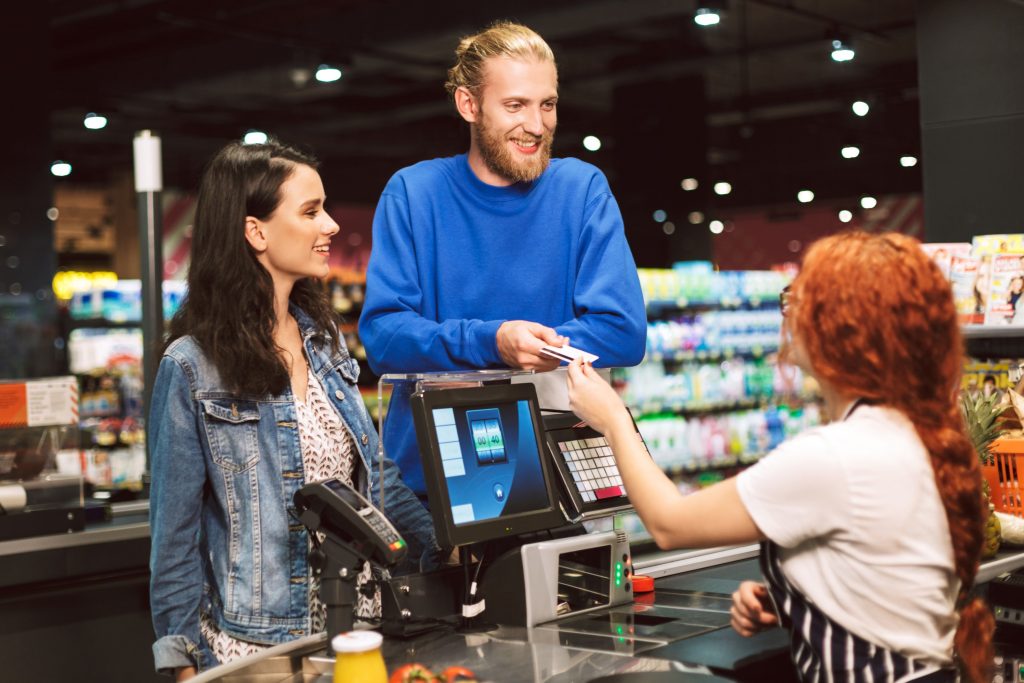
The customer’s primary objective is to pay as little as possible for the right quality products, and businesses aim to maximize revenue and profit. An optimal price is a middle ground for both of these objectives. Katherine Paine sums up the “why” of price optimization splendidly, “The moment you make a mistake in pricing, you’re eating into your reputation or your profits.” Harvard studies have found that a 1% improvement in your pricing can add up to 11% to your profits!
The success of a pricing strategy depends more than just margin (selling price minus cost). External factors like competition, weather, seasonality, events, operating costs, inventory availability, and other macroeconomic conditions play an important role. Channel is also a key factor. To optimize the price for even one product, you need to look at every factor with a clear lens. Before we dive into how retailers do it today, it is essential to understand the fundamentals and evolution of price optimization in retail.
The evolution of pricing – from pre-2000s to 2020s
Multiple changes have happened in the way we price, and some of them have occurred over time. In the last 20 years, data science has unlocked the ability to understand customer demand, which has been a massive benefit to changing how pricing is done.
Pre-2000s
Before the 2000s, retailers were primarily concerned with two major factors – how their competition was pricing (Competitor-Based Pricing) and what margins they wanted to earn. This gave rise to Competitive Indexing and simple Margin-based pricing.
Considering the complexity of relationships that exist among items within the same category, and different ones, it was quite challenging for pricing specialists to keep track of costs and prices while still meeting their objectives. In addition to complex relationships within categories, changing market conditions and dynamic consumer behavior only furthered these challenges.
For instance, consider the pricing for soda products. These are some of the first questions that a retailer would aim to answer: How should I differentiate prices between cases of 6, 12, and 24 packs? What would influence the size of packaging the consumer will buy – how much one consumes, who is being served, or the size of one’s family? How does the demand for cans differ from that of bottles? Is the pricing encouraging customers to stock up (pantry load) or purchase more, and drink more?
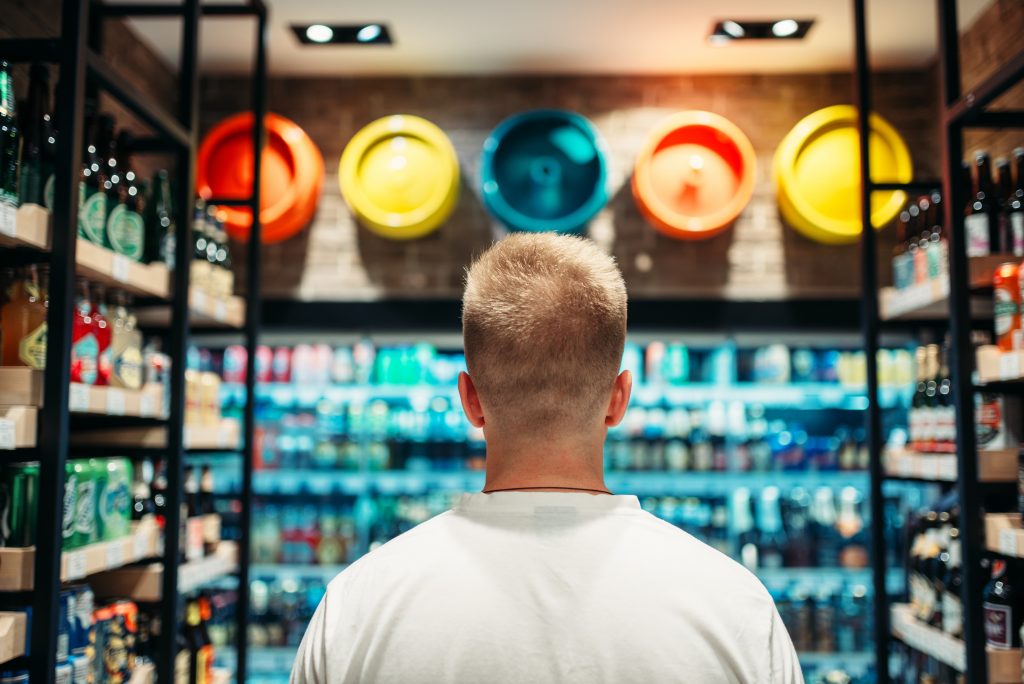
With less sophisticated tools, pricing often turned into a guessing game based on either gut instinct or merely following what competitors were doing. Over time, more sophisticated retailers expanded the scope of pricing beyond simple margin management and followed the competition to accommodate more complex price-determining factors.
Post 2000s – First Generation Price Optimization Systems
Over the 2000s, the data science community began to leverage various algorithms to understand the demand of consumers relative to a price. This work unlocked the ability to quantify the effect that a change in the price had on customer demand. This is called price elasticity.
In these early days of analyzing price elasticity, demand modeling required a large amount of compute power. As a result, most retailers were only able to analyze customer demand on quarterly cycles at the item/category/store level. Beyond infrequent analysis, another criticism of first generation systems is that they would generate recommendations without providing the merchant with an understanding of why a price change was being recommended. Some called this the “black box” effect, and it all too often resulted in user mistrust on the optimized price recommendation.
Another of the most significant problems with first generation price elasticity analysis was that it did not take into consideration what needed to happen in the store to make it a price that could actually be executed. Too many price recommendations were happening too frequently which could overwhelm both merchants and store operations. As a result, many rules and constraints were added later to make prices more consumer-friendly like rounding, and establishing good-better-best brand relationships. These over-riding rules limited the impact of the optimal price determined by elasticity, effectively rendering elasticity-based optimizations as less impactful.
This combination of elasticity and rules in these first-generation price optimization systems clearly had a lot of operational challenges. These systems were usually poorly integrated with core merchandising systems, hard to manage, slow to react to changing customer demand patterns and they were usually unaware of external factors like weather, events, etc.
These challenges drove innovation towards a new generation of price optimization systems.
After 2010 – Second Generation Price Optimization
In 2010, balancing pricing rules and demand-based price optimization was refined with more scalable cloud-based pricing systems. Their answer was to allow the prioritized weighting of factors, which enabled retailers to leverage some benefits of optimization while still managing factors that made a price attractive to a customer (size parity, good-better-best, rounding ending numbers, etc.)
With weighting, retailers could assign a value to factors like elasticity, competitive prices, and margin objectives, which allowed them to prioritize objectives by weight and find the optimized price. This pricing strategy, through its ability to consider a breadth of factors, proved more effective in attracting customers and encouraging purchases.
In this second generation of analyzing customer demand patterns, demand modeling also became more scalable due to the emergence of cloud platforms. Most retailers were now able to analyze customer demand on weekly cycles across all items and categories at every store.
However, despite the huge leap, it still remained hard to manage pricing generated from these systems. They still required too much time from pricing analysts and merchants, and these systems still left little room for rapid price adjustments with changing market conditions and customer behavior as ecommerce channels emerged. In fact, some retailers have been unable to truly leverage such second generation price optimization systems, leading them to move back to rules-based pricing without customer demand-based elasticity analysis.
Retailers who have been stuck with these pain points attributed to First and Second Generation Price Optimization are once again looking to revisit more sophisticated pricing capabilities and are turning to Artificial Intelligence (AI) powered innovation.
How can retailers price better today?
Today, try buying something online from one of the ecommerce leaders and you will see how dynamic pricing has helped them capture considerable market share and stay profitable.
Pricing today is highly competitive, responsive, and sometimes even personalized. Retailers continue to find ways to make it as dynamic and real-time as possible. At a high-level, retailers do it by first identifying KVCs (Key Value Categories) and KVIs (Key Value Items) with inputs like transaction and basket data, shopper price perception data and, of course, merchant judgment.
After identifying KVCs and KVIs, retailers incorporated a combination of multiple forces to arrive at the optimal price for a product as per a McKinsey Study:
- Consumer Demand: This includes factors such as, elasticity, customer-segment product preferences, and in-store and online behavior
- Competitive Positioning: This includes competitor strategies, price comparisons with key competitors, market share trends and competitor price structure
- Internal Economics: This includes actual vs. targeted margin and role and intent
- Category Dynamics: This includes total category growth, current, and projected supply, and demand & category profit pools.
The below diagram helps understand how these forces are weighted for KVIs vs. other background items:
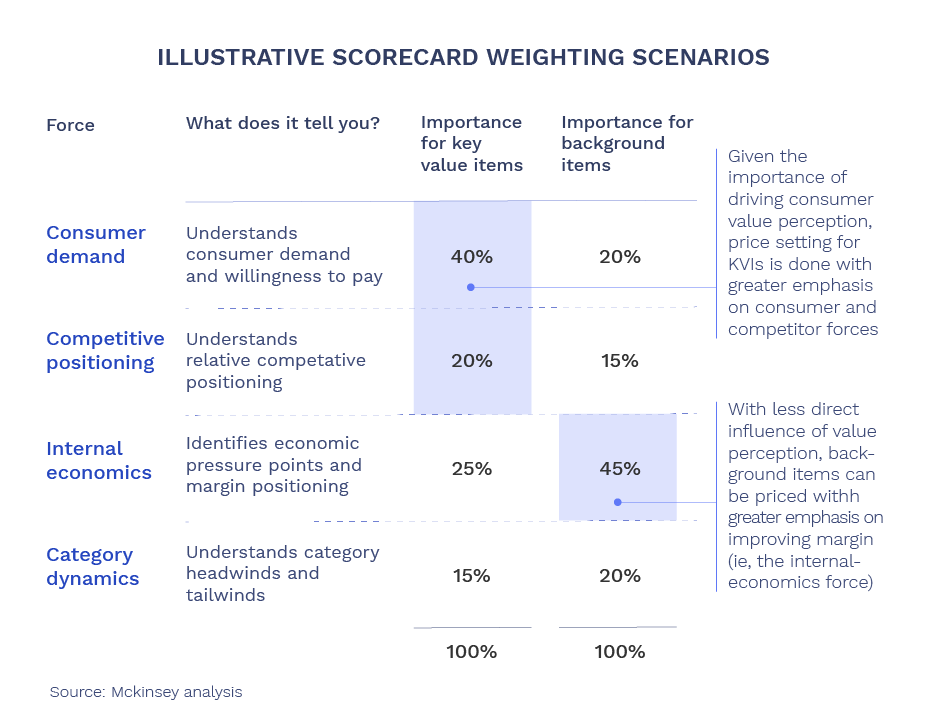
While many retailers can somewhat optimize the pricing with this strategy, what impacts growth for them is speed-to-insight along with a high dependence on analysts, and centralization of critical data to only a few individuals in the team. Human errors could amount to millions of dollars in lost revenue and profit opportunity, since identifying key drivers could take no less than weeks.
AI-powered Price Optimization Systems aim to remedy this with faster speed-to-insight and unparalleled accuracy. But why are retailers still uncomfortable with the quick adoption of such systems? Is there a disconnect between what they need and what AI-powered Price Optimization systems have to offer? Let’s review the problems and solutions one by one.
What do retailers need from their price optimization systems today?
Despite multiple takes on pricing over the years, retailers today are still fairly out-of-step with their customers. As per a RetailWire article, only 20% of retail executives think consumers believe prices are going up online and in stores. Consumers see this differently, with 51% reporting online prices increasing and 60% saying that they are paying more for in-store purchases.
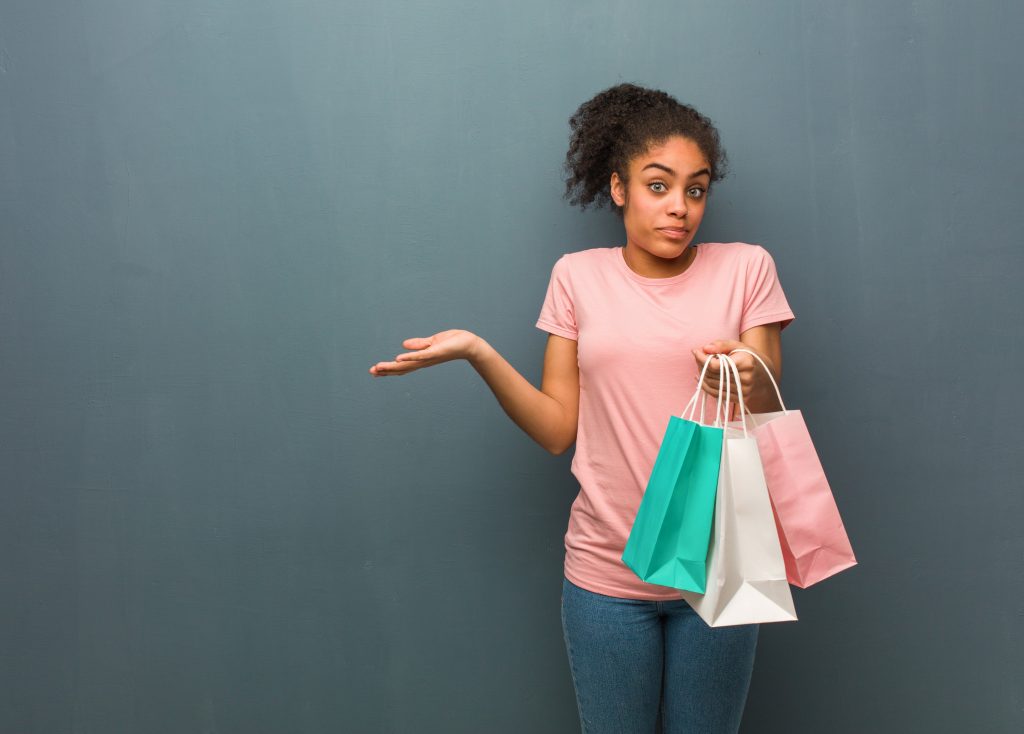
This disconnect is a strong indicator that what worked yesterday is clearly not working for retailers today. Due to legacy practices, this knowledge gap between what retailers think consumers need vs. what consumers actually need is only going to get wider.
Retailers today need a solution that delivers beyond existing practices. While price optimization systems are clearly the answer, many retailers have often found over the past decade that these systems are usually not explicitly designed for their needs, and are too slow, expensive, and complicated.
Here is what retailers today need from their AI-powered price optimization systems:
It should be able to account for complex relationships between items.
An item’s price depends on factors like the pricing of complementary and substitute items, different channels (eCommerce and in-store) etc. There are too many items in stores today to have someone manage these relationships, especially with many variables changing frequently. Retailers need systems that can account for all of these relationships when setting the price in real-time.
Say, a customer is purchasing a diet soda. Would they go for a premium or a lesser priced bag of chips? Or, what if they buy seltzer instead of soda? What about the difference between a can and a bottle?
Changing the price one item (such as a case of soda) could affect multiple other products. Retailers need a system that can handle this 360-degree optimization.
It should be able to provide a holistic solution.
Many solutions that exist today do not account for eCommerce, which is one of the most significant determinants for a customer. For some items, it is very likely that when a considerable spend is involved, your customer is looking up the price of the item online to run a quick comparison check. Additionally, for other items, retailers have the opportunity to charge a premium for items online due to the convenience of eCommerce.
Over the past few months, we witnessed eCommerce become the center of customers’ lives with social distancing. Most pricing systems were rendered ineffective at anticipating and reacting to online demand and price sensitivity.
It should be autonomous, accurate, and reliable.
Say, even if a pricing system were to claim to do all of these things, it could still miss the mark if it was too slow with ingesting volumes of data or its forecasts were inaccurate. Imagine the frustration of an analyst if a solution that only partially works. On top of that, imagine the business owner’s frustration when despite so much time and money investment, their pricing is nowhere near competitive.
Notice the mention of an analyst above? Another common issue that bothers retailers is: if I need analysts to get the work done, why do I need a pricing system anyway?
Retailers today need pricing systems they can trust – systems that can handle data from disparate sources to help them make decisions with real-time data without analysts’ intervention.
Myth: Today’s systems aren’t capable of delivering what retailers need
Believe it or not, AI-powered Price Optimization Systems are capable of meeting retailers’ expectations, while also going a step further to help them understand what’s happening, why it’s happening, and what they can do about it. These systems are commonly referred to as AI-driven, Autonomous Pricing Systems.
A combination of descriptive, diagnostic, predictive, and prescriptive analytics allows these systems to forecast customer demand, set the optimal price, detect anomalies in a matter of seconds and back every decision with real-time data without the intervention of an analyst — a retailer’s dream come true!
Here’s what AI-driven, Autonomous Pricing Systems have to offer today:
- Multichannel: Processes millions of data points in seconds, accounting for data from all online and offline sources. Each channel is evaluated separately.
- Autonomous: It self-learns and self-corrects – turning itself into the only data analyst you will ever need. Pricing is automatically tuned to be customer-centric by machine learning algorithms.
- Accessible by EVERY decision-maker: Say goodbye to a high turnaround time from your data analysts. Get direct access to any data you need in seconds, with a Google-like conversational experience
- Accurate and Reliable: Delivers pricing you can rely on. In addition to accounting for all of your data sources, it processes all of this data in real-time to deliver pricing optimized for today
- Actionable insights and recommendations: Review how your stores are performing with optimized pricing anytime, every day. Your Price Optimization System will provide actionable insights on what’s happening, why it’s happening, and what you can do about it.
- Effortless integration with existing tools and softwares: Already invested in different data integration tools? Protect that investment by effortlessly integrating your price optimization system. Customize your dashboard and cut through the clutter with insights that matter the most to you.
Impressed? See what an AI-powered Price Optimization Software can do for YOUR business.
Meet Hypersonix – the world’s first AI-driven AutonomousAnalytics Platform for Consumer Commerce. Hypersonix goes beyond traditional practices to deliver a holistic solution for retailers with real-time insights on what happened, why it happened, and what they can do about it. Top-tier retailers like Smart & Final and Bashas’ have used price optimization systems like Hypersonix and reported revenue growth and higher levels of customer satisfaction within the first few months.
We recently hosted a webinar on Autonomous AI Pricing, demonstrating all of the above capabilities for retailers, which is now available on-demand.
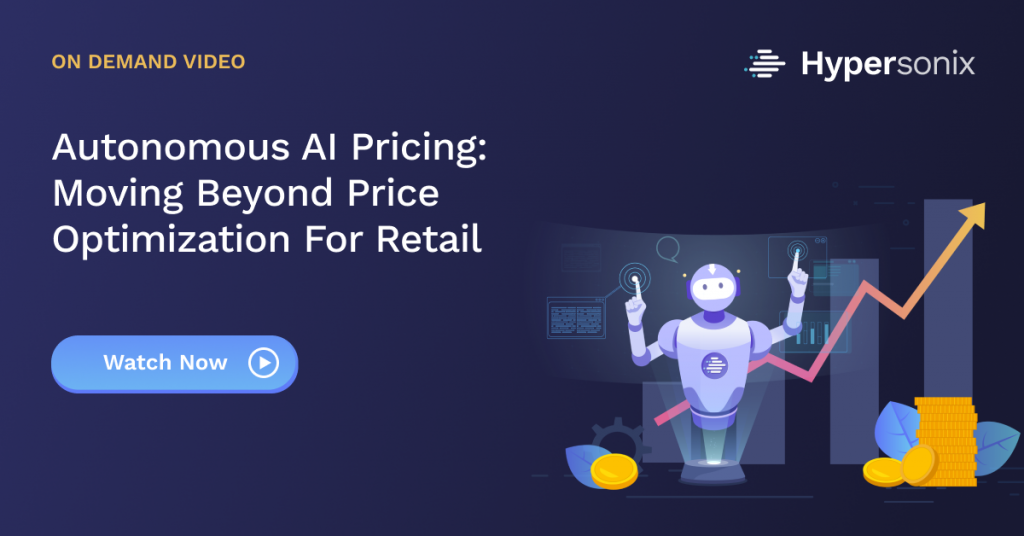
You can also request a demo to connect with a product expert for a personalized demonstration on how Hypersonix can help your business increase revenue growth.