Hypersonix’s Enterprise AI Roadmap: Successful Enterprise AI Deployment
Hypersonix’s Enterprise AI Roadmap: Successful Enterprise AI Deployment
Organizations are sitting on more data than they can imagine, but few have cracked the code to getting value out of their data. To sieve through all that data and perform data analytics at scale, organizations need the power of enterprise AI.
The pandemic has exacerbated this need: more than ever, organizations need real-time insights to help them remain agile and adapt to their ever-changing business environment. Many are eager to accelerate their adoption of AI, but a lack of AI skills, as well as data complexity and silos, remain key barriers to enterprise AI adoption. Even organizations who have experimented with AI struggle with these challenges: this annual trend study on enterprise AI adoption by IBM finds that, year on year, the majority of organizations are still deploying exploratory use cases, but are unable to scale up their AI capabilities.
To advance their AI use cases, organizations first need to tackle the two key challenges: (1) lack of skills and (2) data complexity. While the lack of expertise and skills can be more easily resolved through hiring, training and even outsourcing to partners and ISVs, data complexities are often more difficult for organizations to get around: how do you break down data silos? How do you store data such that it is easily accessible, structure it such that it is easy to use?
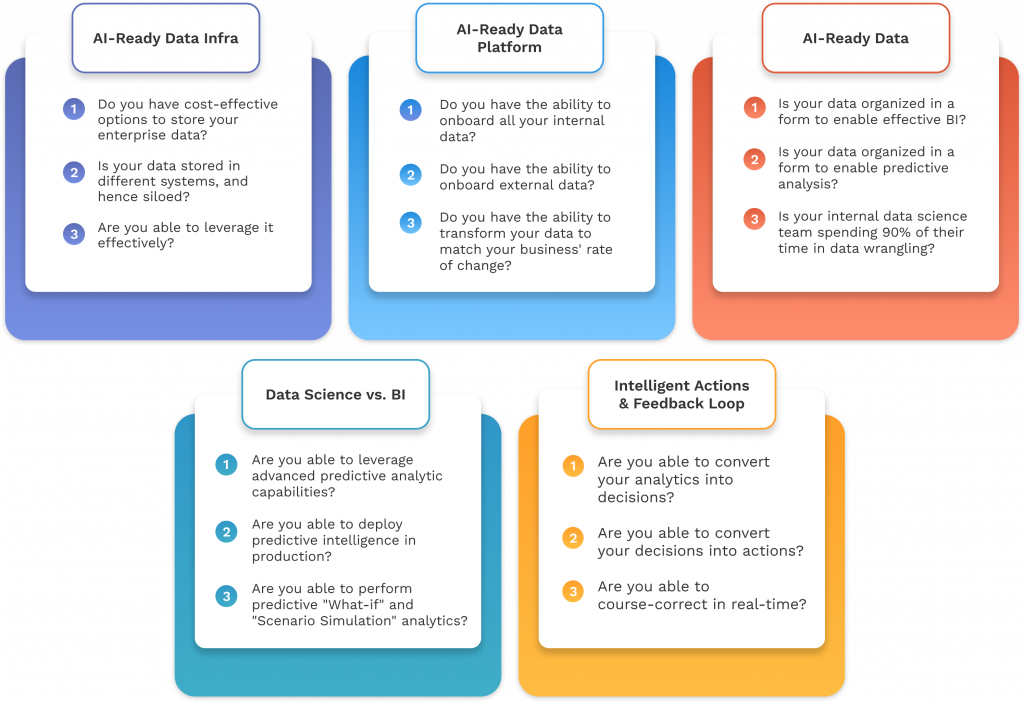
While organizations generally have a well-defined idea of what they want to do with their data, many struggle on the journey there. Hypersonix’s enterprise AI roadmap provides a compass for organizations to stack their data, analytics and AI building blocks in the right order to achieve true data-driven business intelligence.
Regardless of where your starting point is, use this roadmap to orient yourself: did you miss vital milestones that will compromise your AI journey? What’s ahead?
Step One: Build On Top Of An Innovation-Ready Data Infrastructure
AI thrives on data – more specifically, it needs clean data to run effectively. However, legacy ways of operating have left organizations with data that is still stored in disjointed systems, even if they have since reorganized and modernized their operations. This disconnectivity throttles ML, and breaking down data silos is a necessary first step for all organizations on their AI journey.
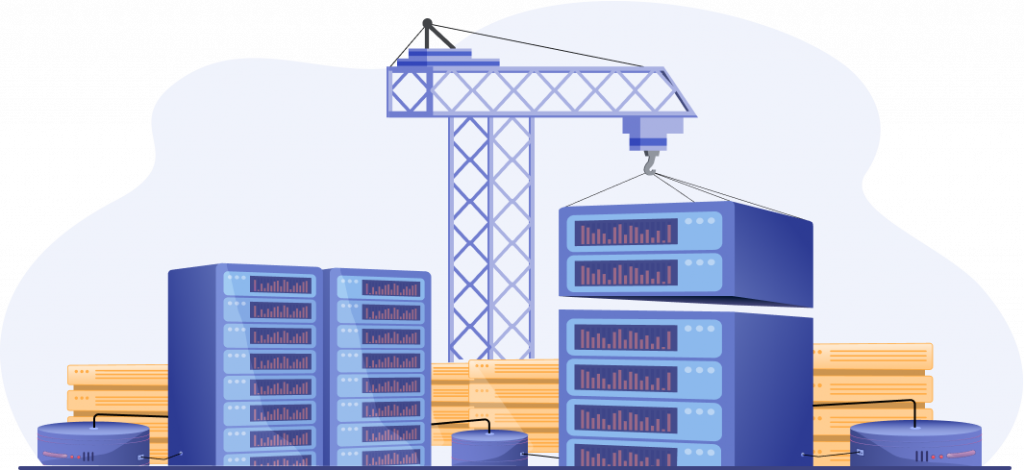
Organizations who have not begun their digital transformation or AI journey may find that, as they start out, much of their historical data is still stored on expensive, legacy hardware servers that lack the connectivity and scalability that AI requires. To progress, organizations need to move their data onto more “innovation-ready” infrastructure that meets AI’s need for speed and flexibility. All newly generated data should also be stored on these modern infrastructure.
Step Two: Manage Your Data With The Right Platform
In many cases, organizations would have introduced some new data technologies (e.g. analytics platforms and data lakes) in recent years, as part of their digital transformation. The problem, however, is that when these technologies co-exist alongside legacy infrastructure, it adds further to data complexities that hamper AI efforts.
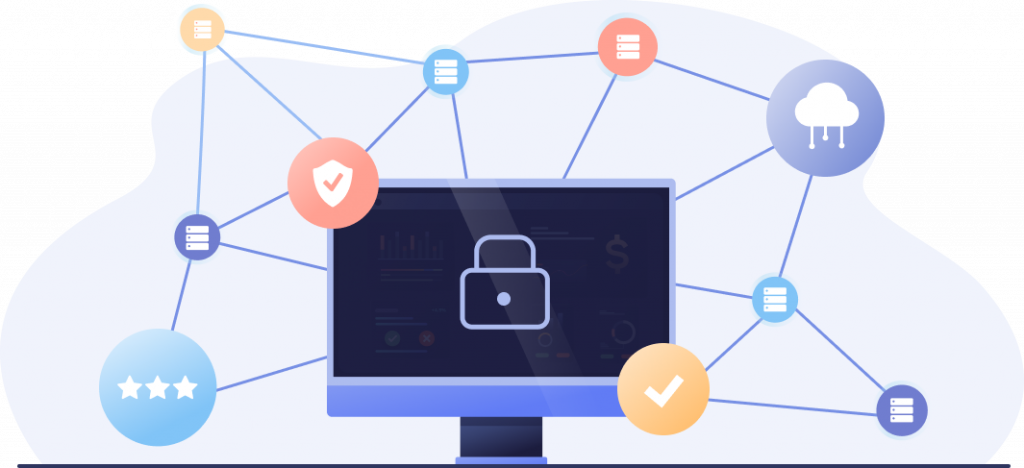
As they start out, organizations may find that their data is organized in largely unconnected, inextensible data warehouses. To head towards a more AI-ready state, organizations require a data platform that not only helps to connect, centralize and manage their thousands of different data sources, but also keep them secure, compliant and accessible.
Step Three: Get Your Data AI-Ready
It is estimated that 80% of data in organizations are unstructured: organizations are knee-deep in unstructured data that hold vital business insights, but left as it is (i.e. unprepped), unstructured data still poses a challenge for most business analytics models.
The next step in getting your data AI-ready involves enriching raw, unstructured data by processing and tagging it to provide some form of structure to it, thereby getting more sense of the information they hold. Ensure that your data is also aggregated across time, location and business categories to keep them organized. Newly generated unstructured data should also automatically be tagged to ensure the data does not regress to its previous state.
While AI can certainly work through unstructured data, having clean, structured data will only improve its effectiveness, and open up exponential new data sources available to analytics models.
Step Four: Move From Descriptive Analytics To Predictive Analytics
Now that organizations have their data in optimal condition for analysis, the focus shifts to how they can utilize this data, and what they can use it for. As they improve the quality of their data, and start integrating previously inaccessible data (e.g. siloed or unstructured data), organizations will start to see that their analytics capabilities will be completely transformed, allowing for more analytics driven by intent. As a start (even if they haven’t performed any of the prior steps, or are still working through them), all organizations should already be performing some form of Business Intelligence on parts of their data – for instance, monitoring key metrics and reporting past performance. But simple business analytics based largely on reporting of historical data is no longer enough.
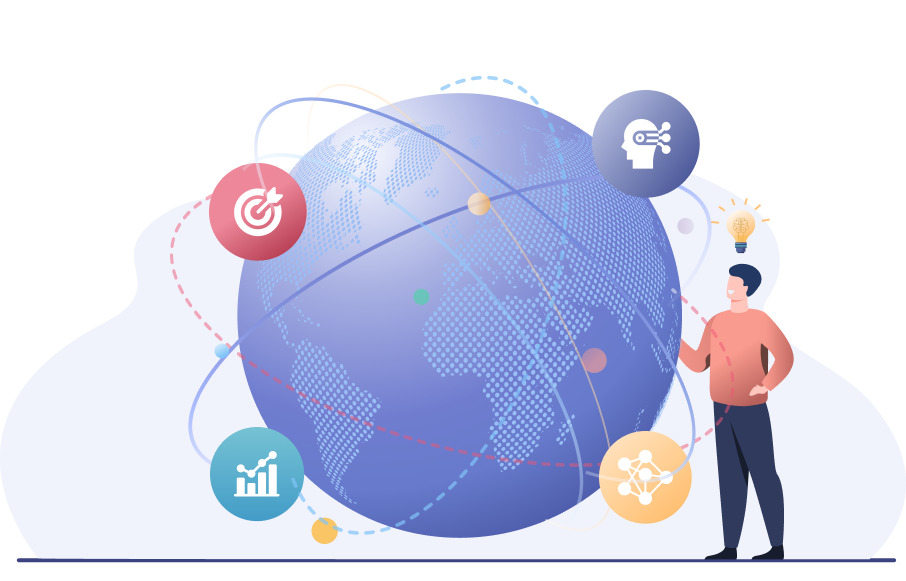
To gain a competitive edge, organizations need to advance towards more sophisticated analytics models that involve forward-looking analysis and predictive intelligence. This includes forecasting and simulation that answer “what if” questions.
It bears repeating that this is only possible if analytics models have quality data to work with: even if your organization has skipped ahead to employing more sophisticated analytical tools, without doing any of the groundwork on your data architecture, it is worth revisiting some of the earlier foundation steps laid out in this roadmap.
Step Five: Create An Intelligent (Data-To-Business) Feedback Loop
The final step in the enterprise AI journey is getting the right insights to the right decision makers. Even now, it’s still common to see organizations and meetings that are guided by static dashboards. These static dashboards often report on historical data that may quickly become outdated and irrelevant.
By drawing from a far wider range of data sources, advanced AI and analytics enable organizations to move towards intent-driven, intelligent reporting dashboards that provide highly contextual and relevant real-time feedback targeted to each individual.
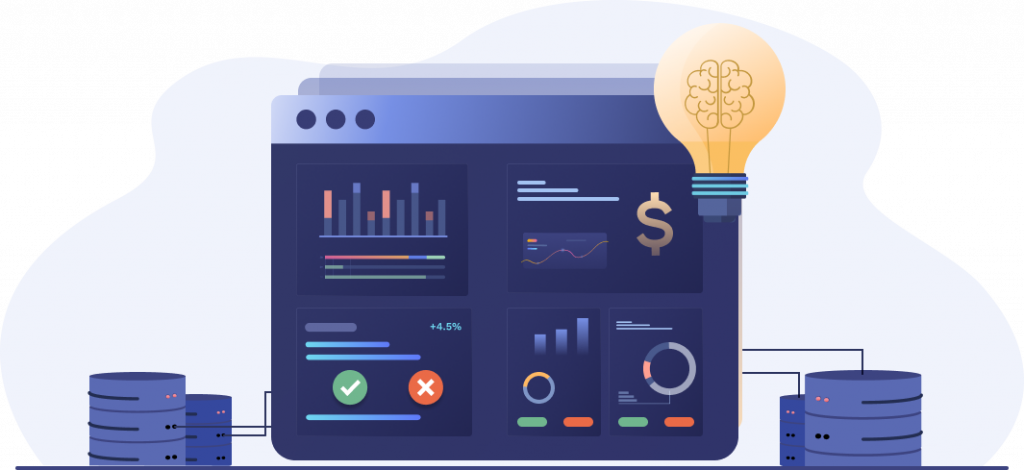
As organizations get accustomed to new ways of working with data, and integrating data feedback into all their decision-making, they can then advance towards even more advanced use of data. In this final stage, with their data, tools and processes all aligned towards the same goal, organizations will be able to conduct simultaneous hypothesis testing (or A/B testing) at scale to help them identify winning strategies as they adapt quickly to an ever-evolving business environment.
***
With the rapid development of new enterprise AI use cases and technologies in recent years, it is no surprise that many organizations want to jump into the game. While the rewards are attractive, organizations will need to put in the effort to make AI work for them – starting with getting their data architecture in place, and then aligning their data with internal tools and processes.
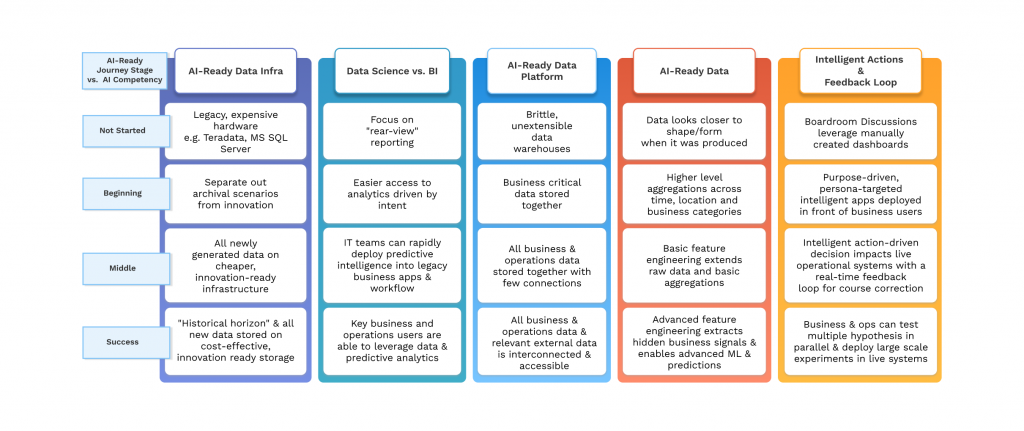
If you are unsure of where you stand in your AI journey today, or if you are headed down the right path on building your data foundations, speak to us.